CadenceCONNECT: The Rise of Photonic Computing
With the growing pervasiveness of artificial intelligence and machine learning (AI/ML), photonics is playing an increasingly important role in enabling AI transformation. We all know photonics is essential for communication, but how about computing? Can it help by not only moving data but also processing data? How? What are its advantages and challenges? Which applications are best suited? How about photonic quantum computing? Learn about the rise of photonic computing, and how it will help unlock the full potential of AI.
We featured presentations from industry and academic experts that will help you tackle many of your design challenges.
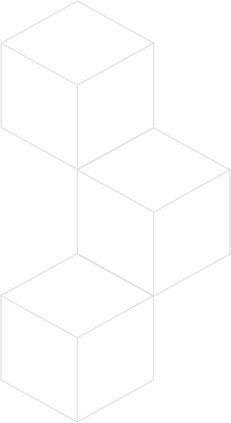
Opening Remarks
Xu Wang, Cadence
Keynote: Neuromorphic Photonic Computing: Classical to Quantum
Artificial intelligence (AI) powered by neural networks has enabled applications in many fields (medicine, finance, autonomous vehicles). Digital implementations of neural networks are limited in speed and energy efficiency. Neuromorphic photonics aims to build processors that use light and photonic device physics to mimic neurons and synapses in the brain for distributed and parallel processing while offering sub-nanosecond latencies and extending the domain of AI and neuromorphic computing applications. We will discuss photonic neural networks enabled by CMOS-compatible silicon photonics. We will highlight applications that require low latency and high bandwidth, including wideband radio-frequency signal processing, fiber-optic communications, and nonlinear programming (solving optimization problems). We will briefly introduce a quantum photonic neural network that can learn to act as near-perfect components of quantum technologies and discuss the role of weak nonlinearities.
Bhavin J. Shastri, Queen's University
Panel: Photonic Computing: Now or Science Fiction?
Moderator: Gilles Lamant, Cadence
Panelists:
Bhavin J. Shastri, Queen's University
Daniel Perez-Lopez, iPronics
Michael Förtsch, Q.ANT
Patrick Bowen, Neurophos
Scalable and Energy-Efficient Tensorized Optical Neural Networks
Optical neural networks (ONNs) are expected to outperform electronic AI accelerators due to their ultra-low computational latency, high throughput, superior energy efficiency, and high parallelism. However, one major challenge for ONNs is the limited scalability. We propose a scalable and energy-efficient tensorized optical neural network (TONN) architecture on HPE’s densely integrated heterogeneous III-V-on-silicon device platform. The tensor-train (TT) decomposition compresses the less important parameters in ONNs and enables the proposed architecture to be scalable to 1024 × 1024 and beyond. Furthermore, the footprint-energy efficiency ((MAC/J) · (MAC/s/mm2)) of the TONNs can be improved by a factor of 1.4 × 104 compared with the state-of-the-art digital electronics.
Xian Xiao, Hewlett Packard Enterprise
Fourier Transform Accelerators Using Integrated Photonics
At Optalysys, we have developed a scalable photonic computing architecture by accelerating Fourier transforms using silicon photonics. The photonic integrated circuits along with electronic feedback logic and re-construction algorithms allow us to compute Fourier transforms of any size and precision. The quad photonic Fourier transform cores form the basis of our QSFP/OSFP pluggable products, as well as chiplets with densely packed photonic cores for extreme Fourier transform acceleration. The scalable nature of the photonic architecture has enabled us to design hardware accelerators for next generation data privacy technologies, such as Fully Homomorphic Encryption.
Imon Kundu, Optalysys
Photonic Architectures for In-Memory Computing Using Nonvolatile Optical Materials
Photonics information processing strategies offer the unique ability to perform analog computation with ultra-low latency and high efficiency. However, designing compact and reconfigurable photonic architectures that scale well is a challenge. The combination of bistable optical materials (such as phase-change materials like Ge2Sb2Te5) and integrated photonics is a promising approach that enables nonvolatile optical memory on-chip with low drift, compact footprint, and high-speed readout. This talk will focus on using photonic memories—together with wavelength division multiplexing and “in-memory” computing techniques—to enable high-speed matrix-vector operations for machine learning applications.
Nathan Youngblood, University of Pittsburgh
Quantum and Photonic Computing Based on LNOI
Over more than 4 decades we have experienced a continuous growth in calculational power. This performance enables us to make ever-better analyses and predictions using ever more advanced computing methods, most prominently driven by the widespread use of AI. To keep pace with the ever-increasing demands on hardware, both in terms of calculation power and energy consumption, the semiconductor industry began to rely on hybrid computer architectures years ago. This includes multicore CPU architectures and the integration of GPUs. Photonic processors offer an additional degree of freedom to this hybrid computing architecture in the form of quantum computing or photonic accelerators. Photonic approaches to quantum computing have gained momentum in recent years due to the advantageous properties of photonic qubits, such as their resilience against noise, room-temperature operability, and mobility. At the same time, the photonic technology opens chances for other near-term applications, for example in the direction of photonic accelerators for AI. In this talk, I will discuss the photonics approach from the perspective of the photonic platform developed by Q.ANT. Our integrated photonic chip platform based on thin-film lithium niobate offers beneficial material properties for photonic computing. These can be leveraged to implement different architectures, like the Gaussian Boson Sampling, quantum-classical optimization, or photonic matrix-vector multiplication, with promising near-term industrial applications, ranging from logistics to AI acceleration.
Michael Förtsch, Q.ANT
Leveraging Design IP to Scale PIC Complexity for VLSI Photonic Systems
Very large-scale integrated (VLSI) photonics requires tight coupling between many photonic elements with electronic I/O, electronic control, and electronic processing. The stabilization and standardization of photonic fabrication processes in recent years enable an ecosystem of photonic design IP. Creating access to validated photonic design IP means that any photonic development effort no longer needs to re-design, re-tape out, and re-test the same structures over and over again and do it with the knowledge that the design will work and meet requirements. Photonic design IP can be leveraged by any R&D team to either develop de-risked designs or to develop radically more complicated designs, such as what is required by emerging photonic processors.
Matthew Streshinsky, Enosemi
Silicon Photonics: The Foundry and Ecosystem
The prediction of the doubling of transistor counts every 18-24 months by Gordon Moore, popularly known as “Moore’s Law”, has been the mainstay of the silicon fabrication industry for decades. However, there is a view that Moore’s law may be running out of steam. The photonics industry has been on the periphery of this silicon industry juggernaut. Several market trends, such the growth in frictionless networking, virtualization where the processing is done in the cloud and AI's ability to quickly process vast amounts of data has accelerated the demand for data and the transition from electrons to photons. Silicon photonics, a technology that sits on the confluence of the electronics and photonics industry, seeks to take advantage of these market trends and address the performance and economic challenges generated from these trends. A critical part of the adoption and growth of silicon photonics will be a disruptive end-to-end ecosystem - from the foundry to the final product. The foundry has a key role to play in enabling this ecosystem. The presentation will explain the role of the foundry in enabling Silicon Photonics to reach its full potential.
Vikas Gupta, GlobalFoundries
Scaling Optical Compute using Metamaterials beyond Digital CMOS
Patrick Bowen, Neurophos
Delivering Data to the Point of Compute with Silicon Photonics
Package bandwidth requirements for various data-center processing units (XPUs) are increasing exponentially, as ever-larger and more complex artificial intelligence (AI) models are developed and deployed. These processors need low-power, high-bandwidth, and low-latency links not only to other processors in the compute network but also to external memory resources. Optical networks are ideally suited to fulfill these connectivity requirements and several solutions, ranging from front-panel optics to 2.5D/3D integrated silicon photonics, are at different stages of productization. But in all these solutions, the bandwidth accessible to the XPU is limited to its beachfront, since all these architectures bring the optics only as far as the edge of the ASIC due to thermal and area constraints. To fully unlock the potential of optical interconnects and get to the next generation of package bandwidths, the photonics need to go beyond the processor beachfront and deliver data right to the point of compute. This talk presents a full-stack silicon photonics-based platform that provides such connectivity, enabled by very high areal density of the I/Os and exceptional temperature stability of the optics.
Subal Sahni, Celestial AI
Towards a Scalable, Automated Photonic Chip Design for High-bandwidth Applications
The Generative AI Boom of 2023 caused a major interrupt in the status quo for optical interconnects, causing ripples in data center, WAN/LAN and telecom infrastructure. Integrated photonics solutions offer the promise of the desired cost and power savings for the future AI/ML systems that are desired by the industry. However, long cycle times for integrated photonics chips that include physical layout, parasitic extraction, design rule checking, test definition, etc. could benefit from significant automation. In this talk, I'll discuss the direct impact of integrated optics for advanced GPU & Switch applications and where the entire design cycle can significantly benefit from increased automation.
Ashkan Seyedi, Nvidia